Opinion
Analyzing the ethical implications and limitations of using artificial intelligence to replace human jobs
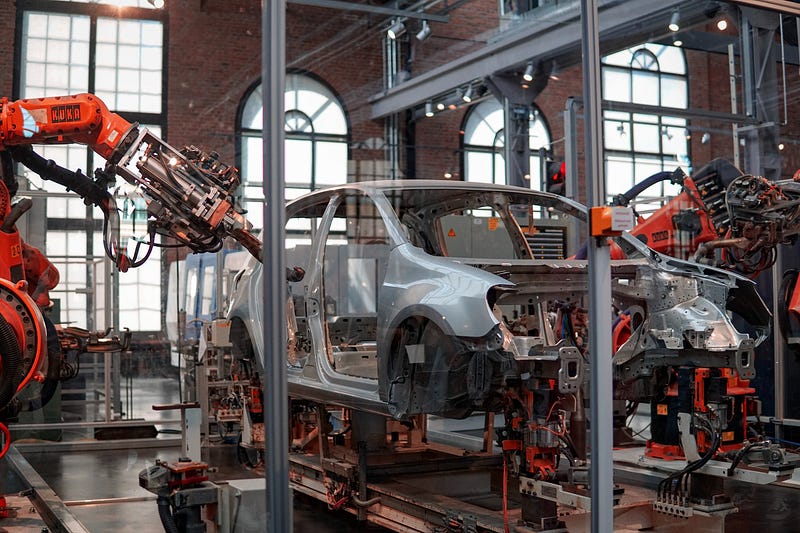
In 2005, Ray Kurzweil, a famous futurist, described the Singularity — a point in time when machine intelligence becomes far more powerful than all human intelligence combined. One idea that is closely tied to the Singularity is the automation of human jobs with artificial intelligence.
The automation of human labor is a phenomenon that we can already see today. Cashier-less stores and restaurants with robot servers are just two examples of automation. Some people even believe that at some point, all jobs will be automated by computer programs. But the real question is, can we truly automate all jobs with artificial intelligence? And even if we can automate many jobs, is the world truly ready for full automation?
The Current State of Artificial Intelligence
The first question we have to ask when considering the possibility of an AI-run society is the current state and capability of AI. There are four types of AI, which can be viewed as different levels of sophistication.
- Reactive AI
- Limited Memory
- Theory of Mind
- Self-Aware
The first two types of AI already exist in today’s world but the last two types are theoretical concepts that will not become a reality until sometime in the future.
Reactive AI
Reactive AI has no concept of memory and is designed to simply react to certain inputs and produce an output. Reactive AI is the simplest form of AI and most early AI systems would fall under this category.
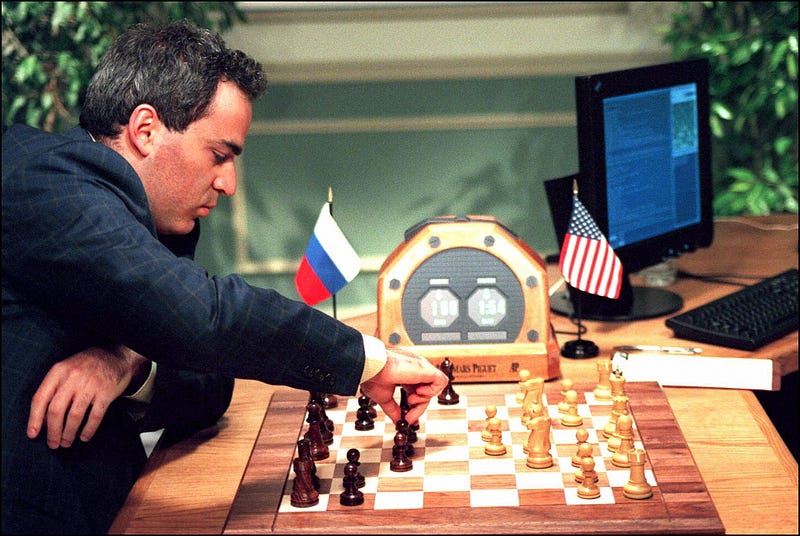
Limited Memory AI
Limited Memory AI is capable of learning from past experience and has a certain degree of memory that allows it to improve its performance over time. Many advances in deep learning such as the use of LSTMs for handling sequential data and the development of reinforcement learning have made this type of AI a reality.
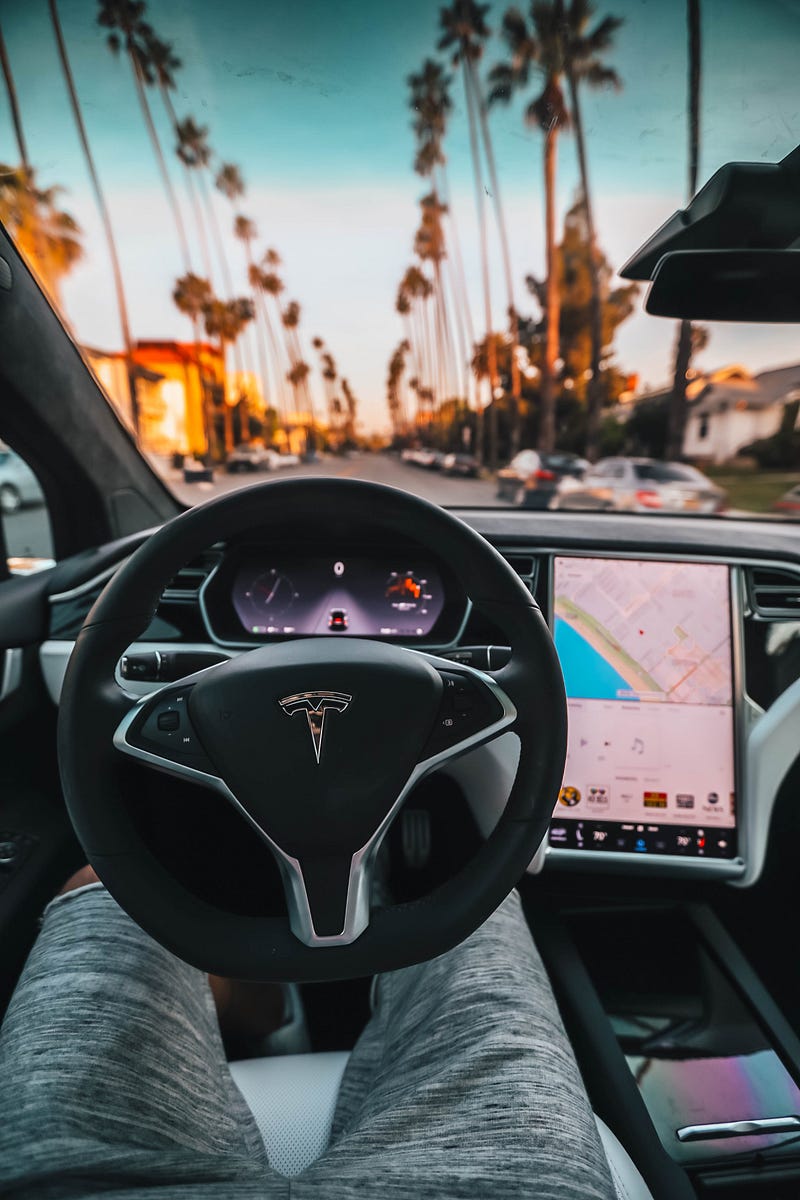
Theory of Mind AI
When machines have the ability to interact with the thoughts and feelings of humans, we will have reached “theory of mind” AI. Theory of mind is a concept in psychology that refers to the ability to understand other people by ascribing mental states to them. When machines have this ability, they will have social and emotional intelligence, which enables them to solve a wider range of problems.
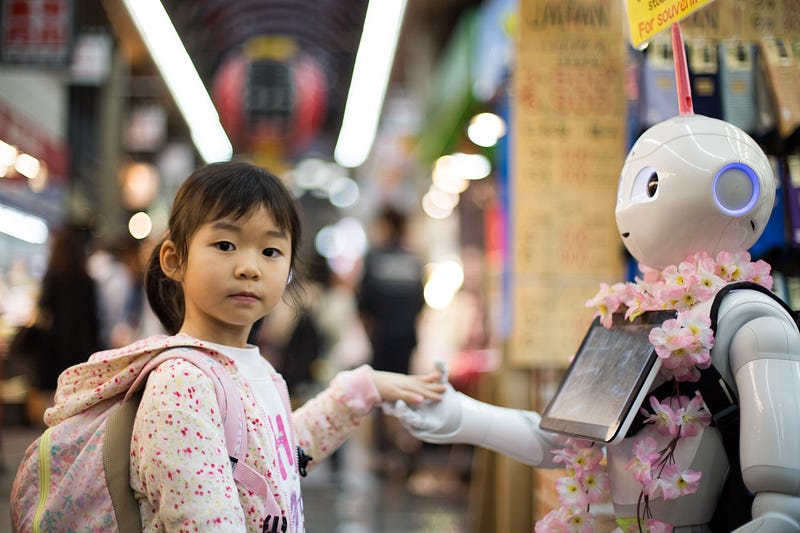
Self-Aware AI
Self-aware AI will become a reality when AI enables machines to achieve self-aware intelligence. At this point, machines powered by self-aware AI will be conscious entities, which raises questions about how machines and humans can coexist.
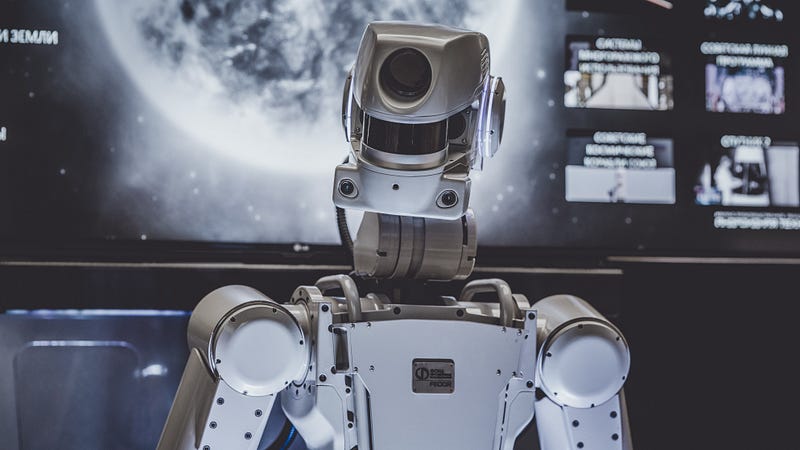
What jobs can easily be automated?
Jobs that involve repetitive steps or predictable decisions can be automated using reactive AI. These jobs often involve manual labor or tasks that involve following clearly defined instructions. Some examples of jobs that fall under this category include:
- Manufacturing and warehouse jobs.
- Manual labor jobs in construction.
- Store clerk and cashier jobs.
- Waste management jobs.
- Manual labor jobs in agriculture (irrigation, harvesting, etc.)
The list above is by no means exhaustive, but the key point here is that all of these jobs involve tasks with clearly defined instructions or predictable decisions that a machine can follow even with reactive AI.
What jobs can eventually be automated?
Jobs that involve more complex decisions based on past events and different situations can eventually be automated but are more difficult to automate than jobs that involve repetitive, algorithmic tasks. A good example of a job that falls under this category is the job of a taxi driver.
There are definitely decision-making patterns that are involved in driving, but drivers need to be able to make situationally-aware decisions. For example, we could train a self-driving car to stop whenever it sees the face of a person. However, what if the car encounters a person whose face is covered? What if the car encounters a dog walking across the road instead of a person? As human beings, we can make these decisions instinctively based on prior experience and what we refer to as “common sense”.
The AI models that power a self-driving car need to be trained to react appropriately to a wide variety of situations and retain some degree of memory regarding past events. In general, jobs that involve more complex, situationally-dependent decision-making can eventually be automated with rigorously trained and tested limited memory AI.
What jobs should be left to humans for now?
Certain jobs require intelligence that is beyond what limited memory AI can provide to machines. Jobs that require social and emotional intelligence will probably not be automated by AI any time soon. In fact, I would argue that these jobs should be left to humans for now. All of the jobs that I have listed below require a certain degree of social and emotional intelligence that exceeds the limits of our current AI systems.
Mental Health Professionals
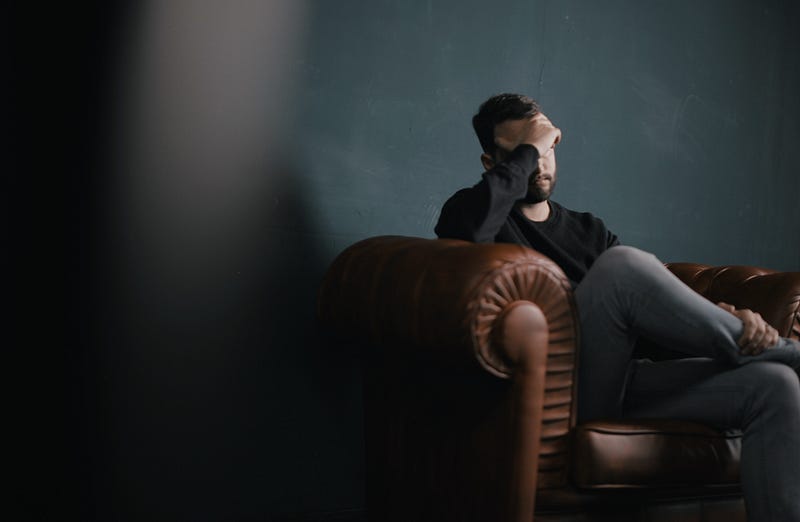
It should come as no surprise that AI, in its current state, cannot effectively automate the jobs of mental health professionals. Therapists, counselors, and psychologists need to be able to understand the emotions and mental states of other people. At the time of writing this article, AI is not capable of understanding human emotions. Some people will argue that large language models such as GPT-3 are capable of human-like conversation, but there is a difference between having a conversation and actually understanding the thoughts and feelings of another person.
Until we achieve Theory of Mind AI, the idea of robot therapists or counselors will not be feasible. In fact, we can rest assured that the jobs of mental health professionals are here to stay for a long time.
Doctors and Medical Personnel
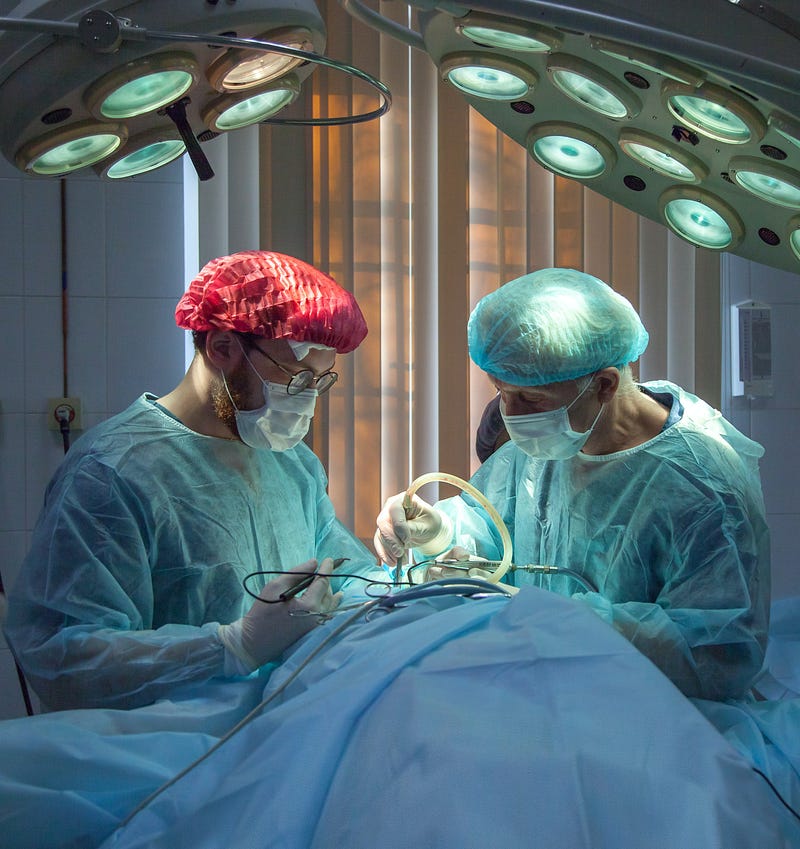
While it may be possible for a machine to perform complex operations such as heart transplants through the use of sophisticated computer vision models, the jobs of doctors and other medical professionals cannot be fully replaced by AI in its current state.
Medical professionals, especially those who work in high-risk environments such as emergency room settings, need to understand the urgency of life and death situations. There are also studies that have shown that empathy is an important part of being a physician. There is a difference between simply curing a disease and actually treating a patient. Treating a patient involves understanding a patient’s concerns and viewing them as a person and not just an illness. A machine driven by limited memory AI can become competent at performing medical procedures, but will always fail to connect with patients on a human level.
There are also ethical concerns that come with using machines to replace the jobs of physicians. For example, if a robot surgeon performs a lung transplant incorrectly and causes serious injury or even death to a patient, then who should be held responsible? Do we blame the doctor who was overseeing the operation? Do we blame programmer behind the robot? Do we blame the robot even though it doesn’t have a conscious mind? These are the types of dilemmas that we will face if we try to automate medical jobs with AI.
Law Enforcement Officers
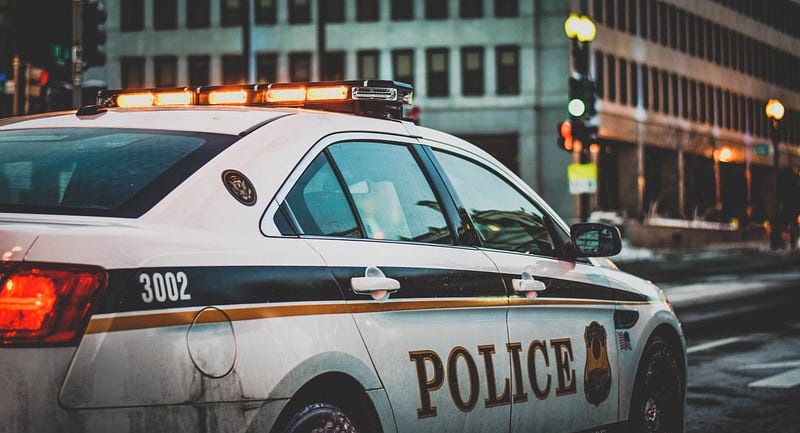
Like the jobs of mental health professionals and doctors, being a law enforcement officer requires both social and emotional intelligence and a level of situational awareness that surpasses the abilities of even state of the art AI. While it is possible to train a machine to fire a gun or detect a speeding vehicle on the road, limited memory AI is not capable of understanding the urgency of situations where there are threats to the safety of other people. For example, limited memory AI may be capable of tracking down an active shooter in a building but fail to understand that the shooter is putting human lives at risk and causing people in the building to panic.
There are also ethical questions that come with arming machines with firearms and placing the lives of people in a community in the hands of machines with no consciousness. If we arm emotionless machines and make them a part of our police force or the military, what is stopping them from becoming ruthless killing machines? Can a community truly feel comfortable and safe if a large portion of the police force consists of robots? If a machine injures or kills a citizen on duty, who should be held responsible? These questions only highlight the fact that we are not ready for AI to automate law enforcement jobs.
Summary
While AI is indeed a powerful tool that can perform many tasks, we need to understand the limitations of AI, especially when it comes to automating human jobs. The most advanced form of AI that exists today, which includes state-of-the-art models such as GPT-3, is limited-memory AI. Limited-memory AI can learn from past data and experiences but lacks the ability to interact with the thoughts and feelings of humans. This ability, often referred to as theory of mind, is required in a wide range of human jobs.
Without social and emotional intelligence, AI can never truly replace all human jobs. Until theory of mind AI becomes a reality, many essential jobs will still be left to humans.
Join my Mailing List
Join my mailing list to get updates on my data science content. You’ll also get my free Step-By-Step Guide to Solving Machine Learning Problems when you sign up!
And while you’re at it, consider joining the Medium community to read articles from thousands of other writers as well.
Sources
- A. Hintze, Understanding the four types of AI, from reactive robots to self-aware beings, (2016), The Conversation.
- L. Greenemeier, 20 Years after Deep Blue: How AI Has Advanced Since Conquering Chess, (2017), Scientific American.
- E. M., Hirsch, The Role of Empathy in Medicine: A Medical Student’s Perspective, (2007), AMA Journal of Ethics.