Exploring the strengths and limitations of this metaphor in the information age.
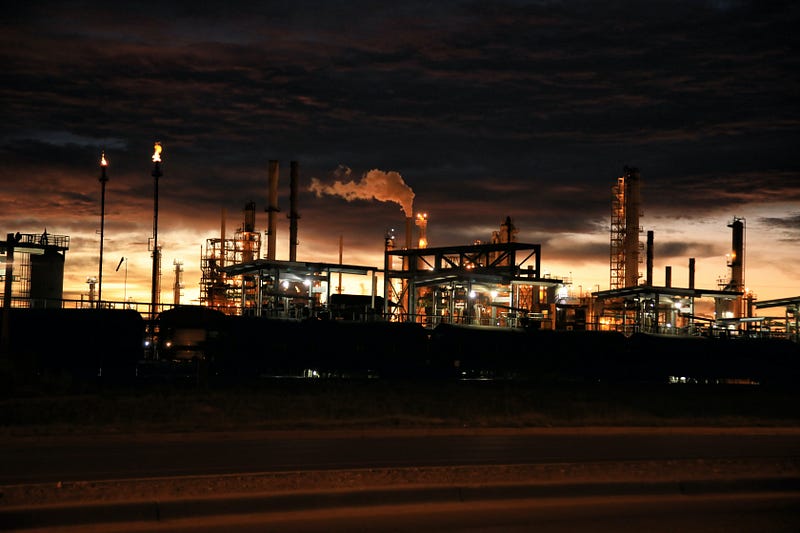
“Data is the new oil. It’s valuable, but if unrefined it cannot really be used. It has to be changed into gas, plastic, chemicals, etc to create a valuable entity that drives profitable activity; so must data be broken down, analyzed for it to have value.” — Clive Humby, 2006
Clive Humby, a British mathematician and data science entrepreneur, originally coined the phrase “data is the new oil” and since then several others have repeated this phrase. In 2011, the senior vice-president of Gartner, Peter Sondergaard, took this concept even further.
“Information is the oil of the 21st century, and analytics is the combustion engine.” — Peter Sondergaard, 2011
Since then, this phrase has become the topic of many articles and has also appeared more often in Google searches as demonstrated in the graph below.

It’s clear that this metaphor has become increasingly popular in the last decade, but is it a sound way of looking at data? Are there better ways of conceptualizing data that can help organizations better understand the role that it plays in the 21st-century business world, especially with innovations in predictive analytics and artificial intelligence? How should businesses approach data as a resource?
What aspects of the metaphor are correct?
The phrase “data is the new oil”, as originally proposed by Clive Humby, does have some merit to it. In a sense, data can be viewed as a resource that is valuable, but only if we can find ways to properly extract value from it.
Data needs to be refined
Like oil, data is only valuable if it is in a usable form. Just as crude oil is transformed into more useful products such as petroleum in oil refineries, raw data needs to be preprocessed before it can be used for analytics. In practice, real-world data collected by businesses for analytics may suffer from some of the following flaws:
- The data contains inconsistent or inaccurate information.
- The data contains missing information.
- The data does not represent the population that it was intended to represent.
- The data is not in a form that is ready for predictive analytics.
Let’s say for example that you run an e-commerce business and want to build a recommender system that uses machine learning to recommend products to customers based on their purchasing habits. You might try to collect information about each customer’s purchasing history and provide them with surveys to obtain additional information so that your algorithm can generate more appropriate recommendations. However, you will have to consider the following questions when collecting and preparing this data:
- What if customers provide incorrect information on surveys?
- What if some customers refuse to fill out the surveys or opt-out of allowing you to collect certain types of information?
- What if you have customers who haven’t purchased enough items for you to make confident recommendations?
- How can you be sure that the data you sampled represents the broader market of customers that you are targeting with your business?
- How can you combine the data from the customer’s purchasing history and their responses to surveys in a structured format that is ready for analytics?
It’s clear that simply collecting raw data isn’t enough for this task. You need to make sure the data is reliable, reasonably accurate, and representative of the market for your products. Even then, you will be confronted with the task of putting the data together in a format that a machine learning algorithm can use to build a recommender system.
Quality data is fuel for analytics and artificial intelligence
To some extent, Sondergaard was right when he said that “data is the new oil and analytics is the combustion engine”. Modern artificial intelligence requires large amounts of quality data to automate tasks normally performed by humans.
Analytics and artificial intelligence are where we finally get to see the real-world value of data. Consider a business inbox with 100,000 emails from customers. This stack of emails seems useless until you use it to generate insights about your customer’s queries and train an intelligent system that automatically categorizes your customer’s emails and directs them to the correct customer support departments. All of a sudden, those 100,000 emails are actually a valuable asset.
It’s easy to give AI and tools like Python, PowerBI, and Tableau all the credit for these business outcomes but the truth is none of it would have been possible without quality data. Quality data is the fuel that drives analytics and artificial intelligence. Just as you can use state-of-the-art engineering techniques to design a car, you can use the most sophisticated mathematical and statistical techniques to design a machine learning algorithm, but at the end of the day, the car is useless without fuel and the machine learning algorithm is useless without data to actually learn from.
Data Requires Infrastructure
Just as oil requires infrastructure for storage and transportation, data requires infrastructure in the form of software and hardware. Any business that wants to maintain data for analytics will need technology for collecting the data and storing the data. This technology can range from on-premise data servers to databases and data lakes maintained in cloud platforms such as Amazon Web Services and Microsoft Azure. The bottom line is that you need a data management system with both a place to keep your existing data and tools for acquiring and storing more data. Good data infrastructure has the following qualities:
- Available — obviously, you should be able to retrieve data from the system in a reasonable amount of time. Especially if you plan to frequently reuse the data for analytics.
- Fault-tolerant — what happens if a machine suddenly fails and the data on it is lost or corrupted? You need a system that can handle events such as these without losing data. This is where distributed computing comes into play in big data applications.
- Cost-effective — data infrastructure that becomes unnecessarily expensive becomes a liability rather than an asset.
Why data is actually quite different from oil
While this metaphor does have its strengths, ultimately data as a resource is very different from oil, and this comparison grossly oversimplifies the nature of data. In order to take advantage of data when solving business problems, we need to understand what kind of resource data really is.
Oil is a finite resource, while data is virtually infinite
While there may be many undiscovered oil reserves in the world, there is a finite amount of oil left on our planet. At some point, we will run out of oil and be forced to transition to other forms of energy. In 2019, the U.S. on average alone consumed 20.54 million barrels of petroleum per day. However, sources from as early as 2018 claim that 2.5 quintillion bytes of data are produced each day globally.
With the number of internet users growing exponentially, we can safely say that data is practically infinite. We will never really run out of data. In fact, we will keep creating more and more indefinitely. This concept leads to the next point.
Oil is consumed, but data is created
When oil is used as fuel it is consumed once and permanently destroyed. Data, on the other hand, is created and does not have to be destroyed even after we use it for analytics. In the information age, everyday human actions generate data every day. Here are a few examples:
- When someone creates a Facebook profile, they are creating data.
- When someone accepts a friend request on Facebook they have created data that Facebook can use for friend suggestions.
- When you watch a movie on Netflix, you are creating data for the movie recommendation algorithm.
- When you buy something on Amazon, you are creating data for Amazon’s recommendation system.
- When you search for something on Google, you are creating data in the form of your search history.
What this means is that data is an asset that doesn’t have to go away and can remain useful for a long time. Technology companies can keep collecting data about customer behavior for years in order to build more robust models that can provide a better experience for customers. Just imagine how much more sophisticated Amazon’s product recommendation system will be after learning patterns in another ten years of online shopping. By updating and improving algorithms with the arrival of new data, companies can turn data into an asset that keeps adding value.
Privacy and ethics come into play when collecting data
So far, data sounds like the ultimate resource for any company. The fact that data is virtually infinite and continues to be created every day seems too good to be true. And truthfully, there are some caveats to this idea. Not all of the data that the world produces is directly accessible to businesses. In fact, a significant amount of potentially useful data may be protected by privacy guidelines and laws. Naturally, there are also ethical concerns that may occur when using data collected from customers. Companies that produce digital products and collect customer data may have to keep the following questions in mind:
- What kinds of customer data can they legally collect?
- What data must remain private if it is collected?
- How can the company protect private customer data from data breaches?
- Is it ethical to use the data collected from real customers for analytics?
These are very real issues and failing to consider them can have serious consequences for companies. Take the famous Facebook-Cambridge Analytica scandal of 2018 for example. In this data scandal, Cambridge Analytica, a British political consulting firm, collected personal information without consent from millions of Facebook users for the purpose of political advertising. This scandal was so serious that it led to the downfall of Cambridge Analytica and caused Facebook’s market cap to fall by over $100 billion in just a few days.
Although there are ethical issues involved in drilling for oil, the privacy concerns that apply to data do not apply to oil. Data is powerful because it is abundant and fuels analytics and artificial intelligence, but with great power comes great responsibility.
What this means for analytics in business
Invest in data infrastructure
Like oil, data is a resource that requires both collection and storage infrastructure to maintain. If you are part of a company that plans to take advantage of analytics or data mining, you need to make sure you have data infrastructure in place to manage your data. Whether your data management solution exists on the cloud or on a physical server that your company owns, you need to make sure it is available, fault-tolerant, and cost-effective.
Collect quality data that is actually useful
The quality of any practical analytics or AI solution is dependant on the data used to build it. High-quality data leads to high-quality analytics. Low-quality data leads to low-quality analytics. If your raw data contains missing or inaccurate information, you may have to refine it until it reaches the level of quality that you need for analytics.
Data can be an asset that keeps adding value
While more oil will not necessarily make a combustion engine perform better, more data has the potential to produce more robust predictive models. Having a system that allows you to collect and store more and more data for training and refining models allows you to turn data into an asset that keeps adding value to your business.
Be aware of the ethical issues involved in data analytics
Data analytics is powerful, but with great power comes great responsibility. Data, especially customer data is a resource that must be handled ethically and responsibly. Always consider the ethical and legal implications of your work if you plan to use customer data or otherwise private data for analytics.
Summary
- Data is similar to oil because it acts as the fuel for analytics and artificial intelligence.
- Like oil, data requires infrastructure in order to collect, store, and maintain it.
- While data is similar to oil, it is much more complex than oil as a resource because it is created and not destroyed and can keep adding more value as more of it becomes available.
- Unlike oil, collecting data comes with issues of privacy and ethics that must be carefully considered.
- While data is valuable like oil, we need to look at it differently when understanding the potential of data as a resource for advancing businesses.
Join My Mailing List
Do you want to get better at data science and machine learning? Do you want to stay up to date with the latest libraries, developments, and research in the data science and machine learning community?
Join my mailing list to get updates on my data science content. You’ll also get my free Step-By-Step Guide to Solving Machine Learning Problems when you sign up!
Sources
- R. K. Ragan and T. Strasser, Big Data: The New Oil Fields, (2020), Credit Union Times.
- L. Adamson, Is Data the New Oil? , (2019), LinkedIn Pulse.
- Wikipedia, Facebook–Cambridge Analytica data scandal, (updated 2020), Wikipedia, the free Encyclopedia.